First-hand insider knowledge: CADFEM Management on AI Trends in Digital Engineering
Selina Popovic
09.04.2025
KI-Trends im Engineering
In recent years, artificial intelligence (AI) has evolved from an innovation promise to a real driving force – at least for companies that have already recognized its potential. Today, AI technologies enable numerous applications in engineering, ranging from the optimization of simple processes to complex simulations. However, the limitations of this technology are clear: AI is only as good as the data it is based on and the models that drive it. We asked our management about this mega-trend and wanted to know what we can expect in 2025.
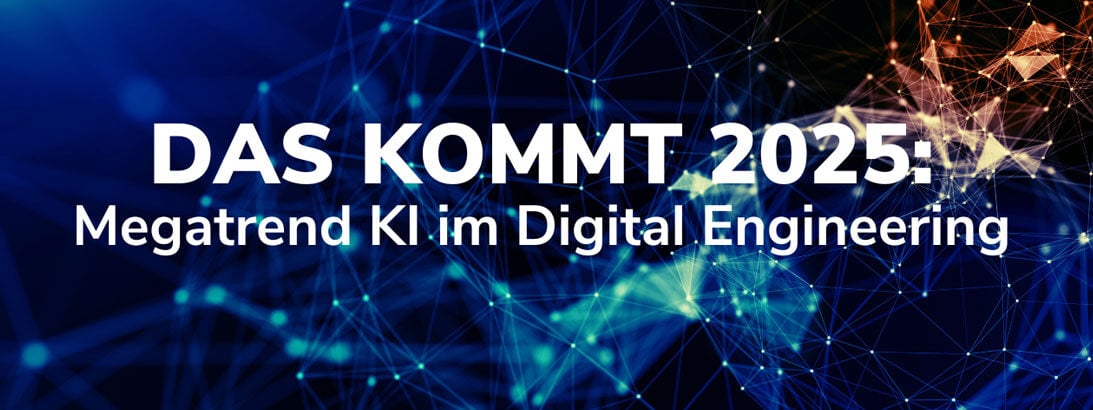
© CADFEM Germany GmbH
Matthias Alberts, Managing Director CADFM Germany GmbH | © CADFEM German GmbH
Matthias, where is AI already being used by our customers and in industry?
Matthias Alberts: Anything that is data-driven and has sufficient data available can be utilized by AI. ChatGPT is certainly the most well-known example. There's also Microsoft's CoPilot, which helps with day-to-day office applications. With this tool, graphics are created using generative AIs, providing enormous support for marketing. AI is even revolutionizing education in schools.
A few years ago, "Big Data" was a hot topic, and everyone wanted to extract as much knowledge as possible from existing data. That’s exactly what is happening now with AI. For businesses, these capabilities are valuable for internal administrative processes and are already delivering significant benefits. Most AI models will incorporate company knowledge, making them a highly valuable and protected asset—potentially a crucial competitive advantage.
In engineering, AI adoption is not progressing as rapidly as with ChatGPT, for various reasons. But here too, as with Microsoft CoPilot, we are seeing software providers using integrated AIs to support users with routine tasks. AI is also becoming a valuable complement to traditional simulation methods.
In the field of digital engineering, AI is already being successfully used in companies to predict innovative ideas and support decision-making based on simulation and test data.
We also see our customers using AI, application data collection, and historical data to develop new business models—creating significant value for their own customers.
Ultimately, the question is not where AI is already being applied, but rather how AI can bring the greatest benefit to each individual company.
How important will AI be for the future competitiveness of manufacturing companies?
Matthias Alberts: AI will increase efficiency, democratize knowledge, and enhance the innovative power of engineers.
Companies with AI-based workflows will develop faster and more efficiently, retain knowledge, and make smarter decisions based on simulations, experiments, and company-wide data. The competitive advantage this creates will be immense, putting significant pressure on companies that lack these capabilities.
Beyond AI-powered product development, future customer purchasing decisions will also depend on how "smart" the products are. In the automotive industry, for example, software is already becoming a key factor in buying decisions. We will see similar effects in other product categories, where AI will play a crucial role.
AI-powered development and smart products—what’s missing? Smart services that enable new business models for manufacturers, such as predictive maintenance.
Josef Overberg, Managing Director CADFM Germany GmbH | © CADFEM German GmbH
Ansys is also focusing on integrating AI into established engineering workflows with its solutions. What specific solutions are being used?
Josef Overberg: Josef Overberg: Ansys is using AI to address many areas:
New solutions like SimAI help users utilize data from simulations and experiments, learn from it, and make fast predictions for design variations.
With AnsysGPT, Ansys provides a completely new way to access simulation knowledge quickly and efficiently, further increasing productivity.
Additionally, Ansys continues to integrate AI functions into its flagship products, allowing users to benefit from AI in their daily simulation work—for example, by accelerating recurring tasks.
However, this is just the beginning. AI can support many more aspects of the development process and simulation solutions. We are still at the early stages of AI technology. One of the next steps will be generative AI technologies that can modify or create geometry models and integrate them with simulations.
Will these solutions replace the engineers working with simulations?
Josef Overberg: No. The question, of course, is, "What does AI do, and what does the human do?" But it is clear: AI will not replace human engineers. Instead, it will support them in solving highly complex, multidimensional problems that would be difficult to tackle using traditional methods.
AI solutions bring knowledge, understanding, and efficiency. AI does not replace humans—it makes them more innovative.
Engineers must therefore develop an understanding of AI and learn about the new possibilities it offers. They should also understand the limitations of AI and know how to validate and optimize AI models to ensure accurate and reliable results.
How is CADFEM shaping the AI market in digital engineering with its offerings?
Josef Overberg: Our goal is to help customers integrate AI into their digital development processes and process infrastructure so they can fully and sustainably leverage its potential.
For example, we support real-time applications for digital twins, autonomous decision-making processes, and intuitive workflows that make AI easy to use for specific engineering challenges. Knowledge management around engineering processes is also essential. That’s why we offer solutions to democratize knowledge and ensure its long-term availability for companies.
Last year, we brought PI Probaligence on board to offer our customers AI solutions for extremely fast and accurate data-driven predictions. The PI algorithm STOCHOS combines neural networks and Gaussian processes, achieving outstanding prediction accuracy—even with limited data.
STOCHOS also excels in evaluating and combining diverse data sources, such as measurement data, simulation results, and key performance indicators. Beyond its AI capabilities, STOCHOS offers a key advantage: it can be easily integrated into Python-based workflows on-premises (locally).
Together with PI Probaligence, we are promoting the use of AI in digital engineering in 2025 and expanding our expertise in cutting-edge technologies—particularly for efficient development processes and design optimization.
Optical simulation
Precision & efficiency combined. Find out in our webinars and further information how you can develop your optical designs with maximum precision.
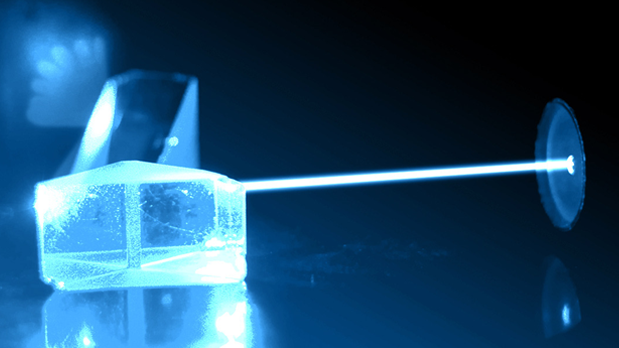
Ansys Startup Program: simulation for startups
Take advantage of the best in simulation technology, right from the start of your business, with a reduced investment.
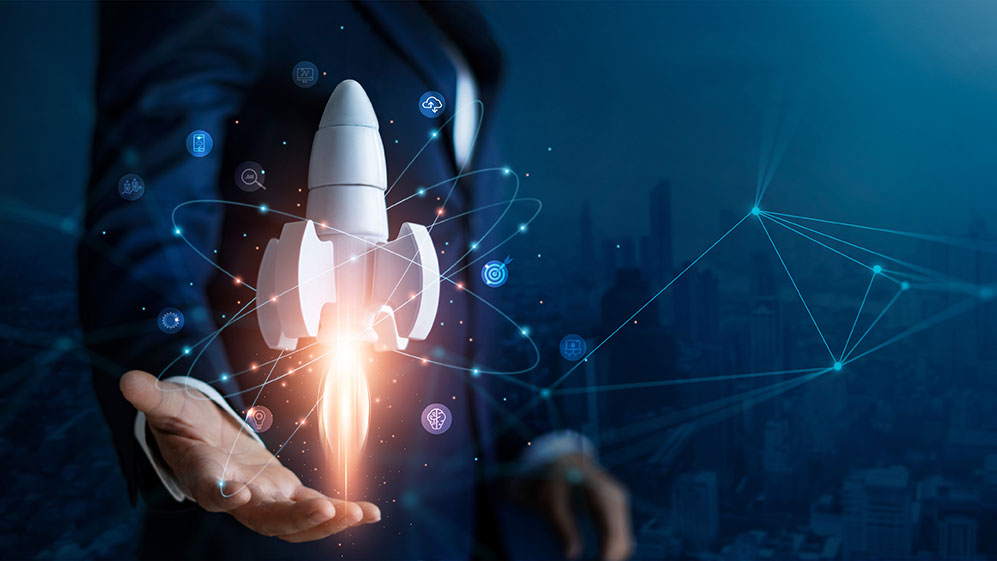
AI for Engineers in Simulation and Product Development
AI and Digital Engineering: Technologies that complement each other perfectly. Bring them together – with CADFEM AI.
![ai-for-engineers-in-simulation-and-product-development-21202[1]](https://blog.cadfem.net/hubfs/02_TOPICS/DE_Corporate%20Newsletter/06-November%202024/ai-for-engineers-in-simulation-and-product-development-21202%5B1%5D.jpg)
Erke Wang, Managing Director CADFM Germany GmbH | © CADFEM German GmbH
How accurate is the AI’s response, and what determines the reliability of the results?
Erke Wang: The accuracy of an AI model always depends on both the quality and quantity of the available data as well as the algorithm used.
Precise predictions are possible when sufficient high-quality data is available, and all relevant influencing factors are considered—especially physical relationships. The more complex and nonlinear the relationships are (or the noisier the data), the greater the data requirements.
The algorithm must also be suitable for modeling these relationships, as simple methods such as linear regression cannot represent non-linear dependencies.
How quickly can an AI be trained if I start tomorrow?
Erke Wang: The time required to train an AI model depends on the availability and quality of data as well as the complexity of the problem. If good data is already available, models can be trained immediately. Simple tasks only take a few seconds, whereas more complex tasks, such as 3D FEM/CFD simulations with variable geometries, may take several hours.
If data is lacking, at least 20-30 simulations or experiments must first be carried out.
Can AI generate data on its own?
Erke Wang: Yes, there are various ways AI can generate data.
One approach is data augmentation, where existing data is expanded through transformations such as rotation, mirroring, or scaling to improve model robustness. However, this can be challenging for specific applications.
Generative AI methods can also create new data, though these often represent variations of existing data.
Another method is AI-assisted geometry generation, similar to topology optimization, but validation through simulations is still required.
What benefits will companies gain in 2025 by integrating AI into their product development processes?
Erke Wang: Companies that leverage AI technologies to learn from existing data create a valuable knowledge base that goes beyond single-use analyses.
By reusing simulation and test data, they can respond more quickly to new challenges and optimize product development.
Additionally, democratizing knowledge allows more employees to access valuable insights, enhancing both efficiency and innovation.
AI helps identify complex patterns and relationships, leading to more precise and intelligent development processes while simultaneously reducing costs and promoting sustainability.
Jürgen Vogt, Managing Director CADFM Germany GmbH | © CADFEM German GmbH
The vast amounts of data that AI needs to process for optimal results are a major challenge for many companies. Will there be a solution for this in 2025?
Jürgen Vogt: For companies to fully benefit from AI-driven efficiency improvements in simulation, the right setup is essential.
Generating simulation-based data, especially in particle or flow simulations, has traditionally been extremely time-consuming. However, this has now changed and will have a significant impact on developments in this field.
The new Ansys solvers, combined with the latest GPU technology, dramatically reduce computation times. This combination enables efficient processing of even the largest datasets and significantly accelerates complex simulations.
Instead of calculating individual operating points, companies can now compute entire characteristic curves—or even multiple sets of curves—in record time. AI and GPUs make it possible to integrate demanding simulations even more seamlessly into the development process and unlock entirely new potential. For example, they allow for the creation of highly accurate models or real-time analysis of large datasets, turning innovation into reality faster than ever.
Matthias Hörmann, Managing Director CADFM Germany GmbH | © CADFEM German GmbH
The integration of AI into existing processes is complex. What challenges do companies face in this regard?
Matthias Hörmann: First, many companies lack a holistic view of their data and processes because isolated systems fragment information. This makes it difficult to consolidate data flows, which are essential in creating a Single Source of Truth—a central, reliable data foundation for AI.
The reliability of the data is another critical point. Consistent and high-quality data is the key to the effectiveness of AI models. Without optimizing data flows and standardizing processes, the potential of AI remains limited.
Moreover, successful integration requires that AI becomes part of the company's digital infrastructure. This not only means structuring data properly but also ensuring the regular maintenance and care of AI models. They must be integrated into continuous data streams and regularly updated with new, validated information to ensure relevance and accuracy.
Ultimately, integrating AI presents an opportunity to drive digitalization by pushing companies to modernize existing systems, standardize processes, and strategically align data management. Only on this foundation can AI reach its full potential and create long-term value.
How do you assess the democratization of AI in engineering?
Matthias Hörmann: In 2025, it will definitely continue to progress, driven by more accessible tools and lower technical entry barriers.
Low-code and no-code platforms enable engineers to integrate AI models into their workflows without deep programming knowledge. This development promotes wider usage as specialized teams can combine their domain expertise with AI technology.
A central trend is AI-driven automation of complex development processes, for example in simulation, prototyping, or quality assurance. Scalable, cloud-based solutions and modular approaches will become more significant, making it easier for companies to adapt AI flexibly to their individual needs.
However, democratization also comes with challenges. Companies not only need to master technical fundamentals like data management but also address ethical and regulatory questions. At the same time, there will be a stronger focus on explainability and transparency in AI decision-making to build trust in the technology.
Significant changes also often bring fears and concerns among employees. How can this be countered?
Matthias Hörmann: Successful AI implementation requires change management to build acceptance and trust among employees. It fosters open communication to reduce fears and misunderstandings and supports the development of new competencies through training.
At the same time, it accompanies the cultural transformation towards a data-driven way of working. Change management ensures that processes are adapted so that AI solutions are efficiently integrated and that AI is seen as a support and not a threat.
By linking technological, organizational, and human aspects, the transition is simplified, laying the foundation for the sustainable and successful use of the technology.
Conclusion
AI will continue to grow into a key technology that enhances efficiency and innovation.
Particularly in the field of Digital Engineering, approaches like Large Language Models (LLMs), Machine Learning (ML), and specialized algorithms such as STOCHOS can showcase their unique strengths in specific application areas. However, humans remain indispensable, as they must validate AI models and understand their limitations.
To ensure that you develop the right approach to AI and simulation in your company, it is crucial to engage with all aspects early on. This requires establishing the foundation within the company, especially in product development: digitalization.
We would be happy to support you in implementing AI-powered solutions to sustainably unlock their full potential – a crucial step towards smarter products and a more digital future.
Ansys HFSS
Industry standard for determining the radiation and transmission behavior of high-frequency electromagnetic fields.
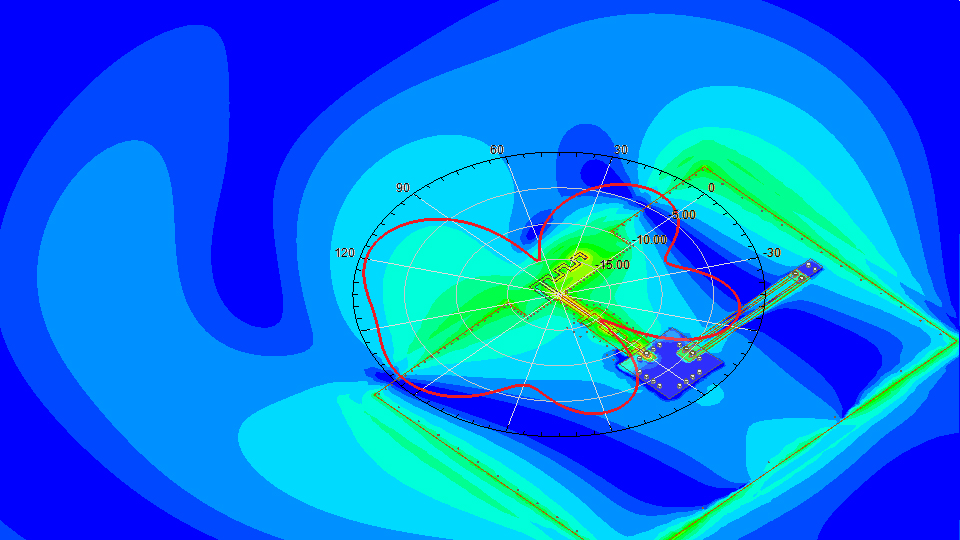
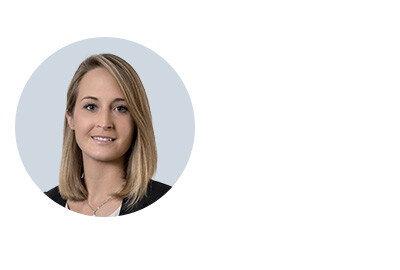