AI is a Management Issue: Management, Implementation, Moderation
Alexander Kunz
08.04.2025
What to look out for when introducing AI methods in engineering
The implementation of new methods such as AI and ML must be well prepared and moderated. They don't work “out-of-the-box” because every organization has different requirements and challenges. Kevin Cremanns, founder of the AI start-up Probaligence and the brains behind the AI solution STOCHOS, has actively supported many implementation projects. In this interview, he explains what managers need to look out for.
Kevin, AI is bringing major changes to the company. Due to its disruptive effect, do you think its implementation is primarily a task for management?
Well, what I and my colleagues are seeing with customers is that a company needs to have an AI strategy and someone in charge who has the big picture in mind. What we also see is that AI cannot simply be pushed through top-down. Someone saying “we're implementing AI now and you're all going along with it” does not work. When considering AI in product development, domain experts and engineers also need to be involved. They need to be convinced by AI and know its value. After all, they are the ones who generate the data and can assess whether an AI model is correct or not. They have to work with it, not the bosses.
For implementation, resources are needed, and their provision is a management issue. For example, the time required for those responsible for dealing with a use case. What else?
We are talking about something holistic here that needs to be managed globally. This also includes providing information and easing the concerns of engineers that they could be replaced in the medium term. Instead, they should see AI as a tool that speeds up and simplifies certain processes, relieves them of repetitive tasks and gives them more time for creative tasks.
We have also experienced the need for leadership in customer workshops, for example when opinions on AI differ between simulation and test engineers. Management has to moderate and make decisions. It is important that management ensures that everyone involved is present in such workshops.
When AI is implemented, employees also need to be appropriately qualified. Does a company necessarily need machine learning experts?
You don't actually need AI experts to use our STOCHOS solution. STOCHOS is a tool for engineers and domain experts. Of course, it doesn't hurt to know a few basics such as which data in which form? Which variables can be predicted with AI? Which are rather unsuitable? In order to develop a feel for this, users should familiarize themselves with the topic.
CADFEM can also support its customers in this. The eLearning on the subject of AI is particularly helpful. With STOCHOS, we recommend building up basic knowledge. However, for many other solutions, especially open source solutions, this will not be sufficient, as significantly more AI expertise is required.
TIP: Hype is Good – But Results are Better!
The industry is at a revolutionary turning point: Artificial Intelligence (AI) is no longer a vision of the future but a tangible competitive advantage. But how can this technology be used effectively in product development? Our eBook not only provides answers but also delivers practical knowledge with real value.
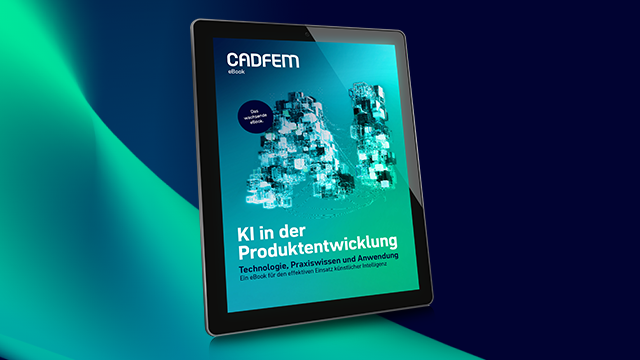
Is there actually a general procedure for implementing artificial intelligence in a company?
Not really. There are always differences depending on the size of the company, for example. In large companies, there is an IT manager, an engineer and perhaps even an AI specialist as well as other technical experts. This allows tasks relating to AI implementation to be distributed. The IT manager knows the computing resources, clusters, cloud solutions, you name it. The engineer takes care of the data and the AI specialist makes sure that the model is trained with the data - on the cloud that the IT expert has set up. If you are set up accordingly, I would definitely recommend interdisciplinary teams.
In smaller companies, it tends to be one and the same person. This person, usually the engineer, has to take care of the IT themselves. They have to be able to calculate the model and operate the AI tool. This is where a tool like STOCHOS really shows how useful it is.
Are there limits to AI? In other words, what are the use cases that are not suitable or that are particularly suitable?
Basically, wherever there is data with more or less deterministic patterns, you can try to build a corresponding model from it using AI. This involves test data, simulation data, production data, robotics control and so on. The company determines what the specific goal or expectation is. But of course AI also has limits, which everyone should be aware of. What an AI tool cannot do, for example, is completely replace a simulation. The data has to come from somewhere. That's why an AI tool will never replace an engineer who understands physics and can assess which data and parameters are needed and whether what the AI says is plausible. AI is simply another tool.
Watch the full interview here: Watch the YouTube video
Ansys HFSS
Industry standard for determining the radiation and transmission behavior of high-frequency electromagnetic fields.
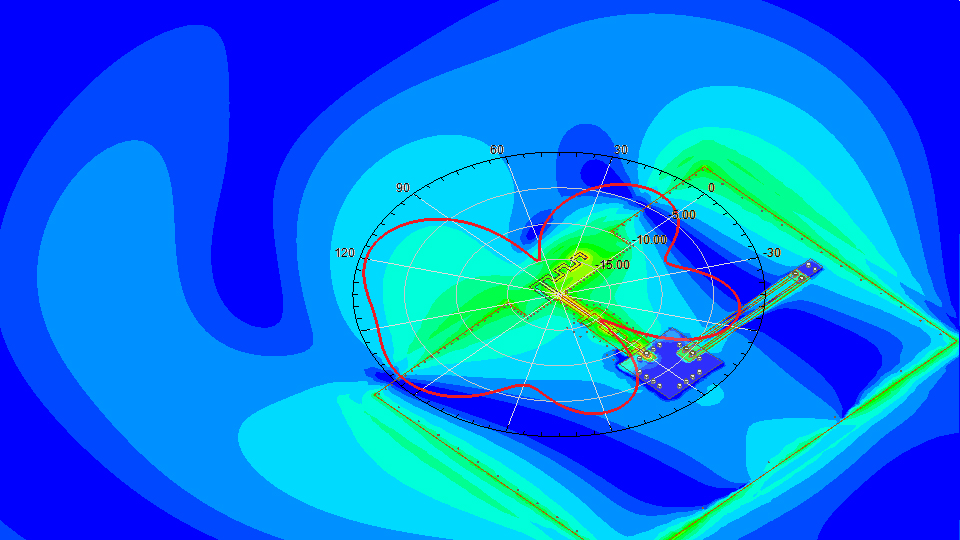
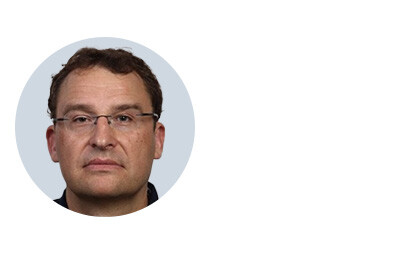
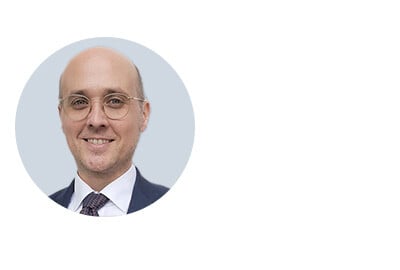